Bridging the Gap: How Data Governance and Data Quality acts as an enabler for Real-World Data Science.
Introduction
The Healthcare and Pharmaceutical industries are witnessing a rise in the use of real-world data (RWD) to generate real-world evidence (RWE) as the RWD data sources like electronic health records (EHRs), Disease registry, wearables, and claims data, offers better information into patient experiences than the controlled setting of clinical trials.
Real World Data holds immense potential to revolutionize healthcare, however this rich data sources also presents a complex challenge in
- Responsible use of Real-World Data
- Ensuring Data Quality
To harnessing the true power of RWD requires a robust foundation built on two critical pillars Data Governance and Data Quality
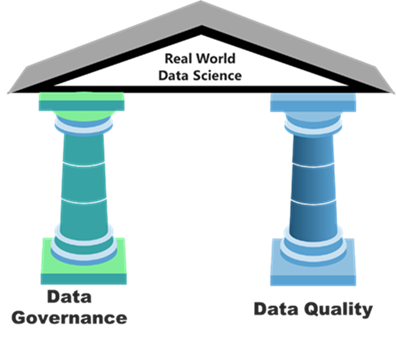
Let us see how data governance and data quality checks act as an essential tool in transforming messy data into clear and impactful real-world evidence (RWE).
1.Data Governance: Building Data Foundation
Data governance establishes set of policies, procedures, and practices that ensure the responsible collection, storage, access, and use of RWD.
Let us see why Strong data governance is crucial?
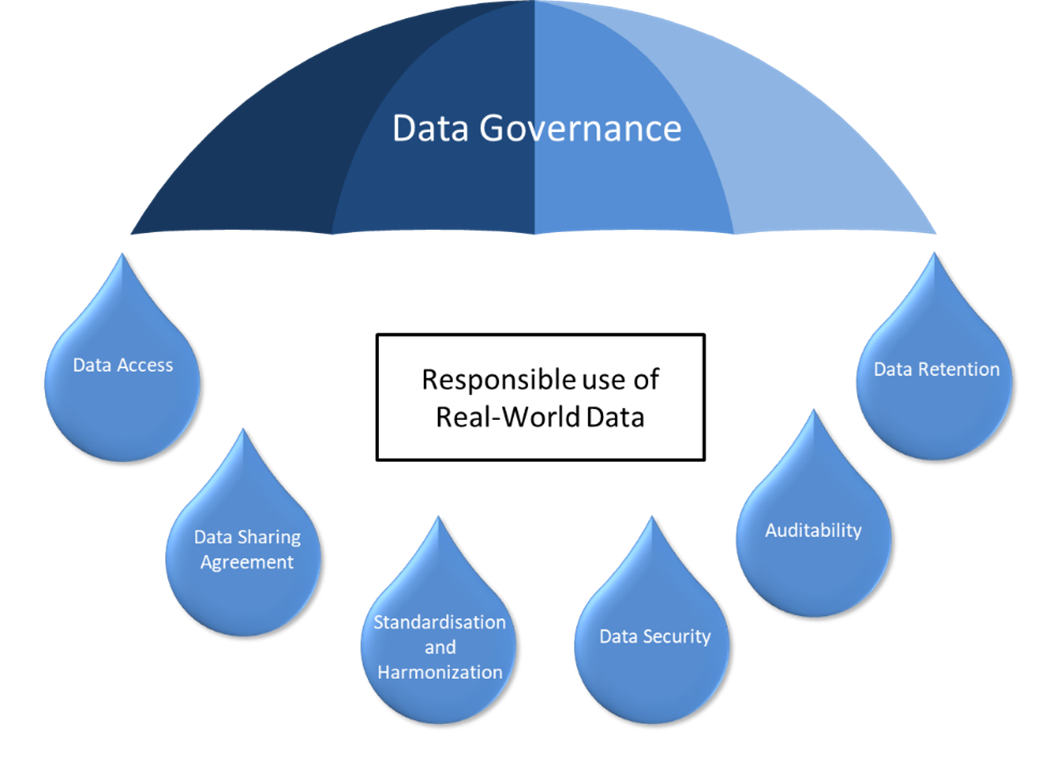
- Data Access: Starting with creating clear protocols which define who can access RWD and for what purposes. This protects patient privacy and ensures data is used for responsible use.
- Standardization and Harmonization Defining common data elements and formats across healthcare institutions is the key which minimizes inconsistencies in RWD collection. This allows for accurate data integration and analysis, leading to reliable RWE generation.
- Data Security Ensuring robust security measures to safeguard sensitive patient information from unauthorized access or breaches. Additionally, it helps to be in compliance with data privacy regulations like HIPAA and GDPR.
- Auditability Creating an audit trail that tracks how RWD is accessed, used, and modified. This transparency allows for accountability and facilitates regulatory compliance
- Data Retention Developing clear policies which define how long data will be retained and under what conditions. This adheres to data privacy regulations and ensures responsible real data management.
2.Data Quality Checks: Ensuring Reliable RWE Insights
High-quality RWD is essential for drawing accurate conclusions about patient outcomes.
Let us see how data quality checks help to ensure reliable insights?
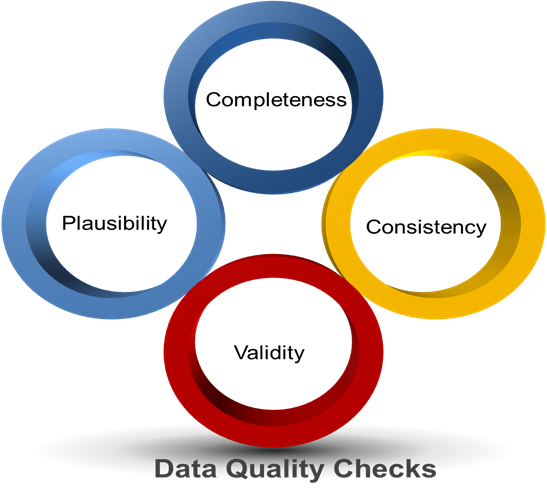
1. Completeness Checks
- Identifying Missing Data: Techniques like data profiling identify variables with a high percentage of missing values (Example: Height (20%) and Weight (10%)).
- Data Imputation: Statistical methods estimate missing values based on existing data patterns. (Example: Imputing missing blood pressure readings based on historical data and other patient demographics.)
2.Consistency Checks
- Internal Consistency: Verifying data points within a patient's record are consistent (Example: Diagnosis of Liver disease aligns with prescribed medications).
- Data source Consistency: Checking for consistency across different data sources (Example: Diagnosis code in EHR database matches the code in a claims database).
- Standardization Checks: Ensuring data adheres to standardized formats and coding systems (Example: verifying diagnoses are coded using ICD-11 standards).
3.Validity Checks
- Format Checks: : Ensuring data types are correct (Example: dates in DD-MMM-YYYY- format).
- Range Checks: :Verifying data falls within expected ranges (Example: Validating a patient's age to be between 0 and 120 years old).
- Clinical logic Checks: : Ensuring data adheres to pre-defined logics (Example: a patient diagnosed with a specific condition shouldn't be prescribed a contraindicated medication).
4.Plausibility Checks
- Identifying Outliers: Employ Statistical methods detect data points that deviate significantly from the norm (Example: unusually high dosage for a specific medication).
- Investigative AnalysisInvestigating if the patient has unique medical conditions or factors that might justify the outlier (Example: Rare genetic variation influencing drug metabolism).
Conclusion
Overall, strong Data Governance ensures RWD is collected and used ethically (Responsible use), while, Data quality checks which guarantees the insights derived from it are accurate and reliable. These two aspects are crucial for unlocking the true potential of RWD which ultimately paves the way for a data-driven future of improved patient care and targeted drug development.